We have entered the Age of Artificial Intelligence and Generative AI is developing at a rapid pace and becoming integral to our lives. According to Bank of America analysts, “just as the iPhone led to an explosion in the use of smartphones and phone apps, ChatGPT-like technology is revolutionizing AI”.[1] Generative AI is changing every aspect of our lives including education, healthcare, entertainment, customer service, legal analysis, software development, engineering, manufacturing, and more.
A key Generative AI performance breakthrough is the transformer: a machine learning architecture that better understands context. These transformers enable large language models (LLMs) including OpenAI ChatGPT and DALL-E, Microsoft Bing/Copilot, and Google Bard to create human-like text interaction, artistic images, and expert-level personal assistants – capabilities that seemed futuristic just months ago.
The race to deploy and build on these tools is predicted to drive robust demand for processors, memory, and connectivity chips that are used for training and running these AI models. This represents a significant opportunity for savvy tech companies and investors. According to a May 2023 report by Next Move Strategy Consulting, the global AI chip market is expected to increase from $28.83B in 2022 to $304.9B in 2030 and grow at a CAGR of 29.0%.
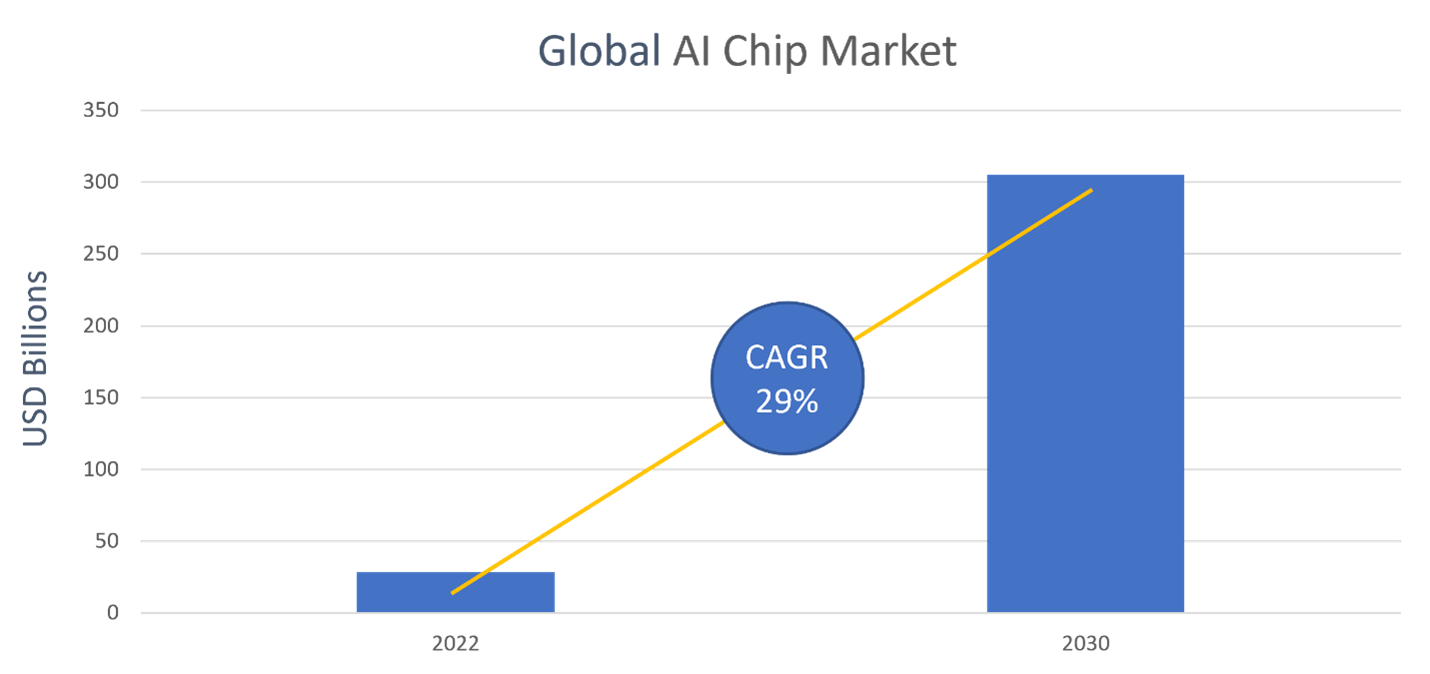
Source: Next Move Strategy Consulting
AI Compute Outpacing Moore’s Law
In order to achieve great performance, general LLMs require extensive pretraining, using large datasets. For example, ChatGPT was reportedly trained on 570GB of text, taking 34 days despite running on 10,000 GPUs.[2] [3] As models become multi-modal (able to process text, imagery, and audio), training data size for general LLMs may increase.
The computational power required to train large models on big datasets is rapidly outpacing Moore’s Law. While single GPU performance is increasing by about 2x every 18-24 months (Moore’s Law plus architectural improvements), training dataset and AI model size are growing by 10x and 35x, respectively, in the same time frames.[4] If the training needs to be completed in a reasonable number of days, the power of dozens or even 1000s of GPUs may be required.
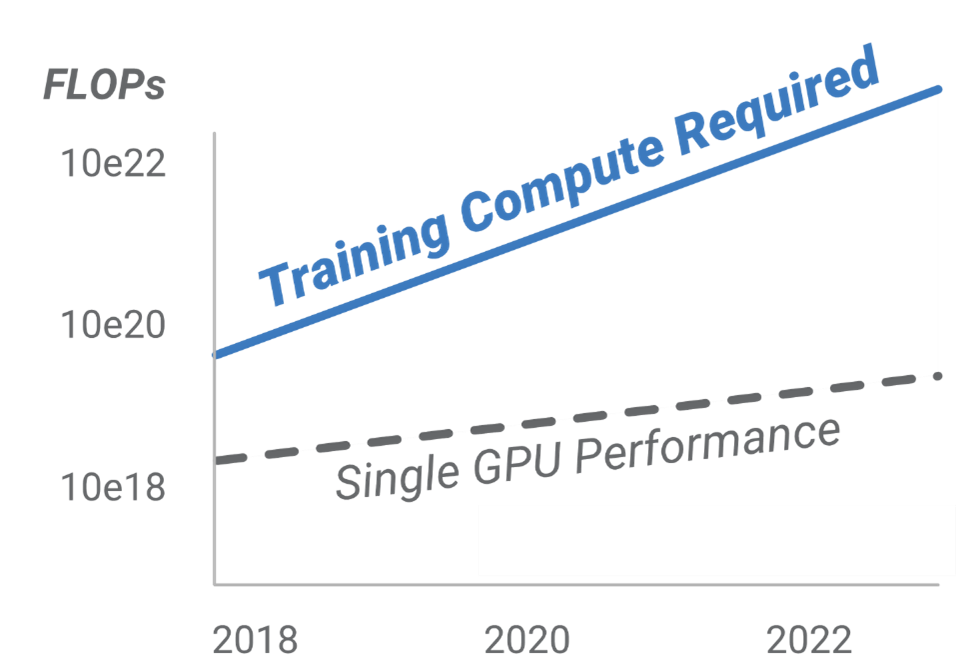
Source: Sevilla, Jaime, et al. “Compute trends across three eras of machine learning
Fortunately, transformer training lends itself to being parallelized as it can run on multiple GPUs. Using this parallel processing technique allows Generative AI models like ChatGPT to learn a great deal about our world in a matter of weeks.
Disaggregated parallel compute architectures, however, still require processing nodes to share vast amounts of data in real-time with each other. This creates a bottleneck at the interconnects between processing as well as memory components. These connectivity bottlenecks become more challenging as hardware scales and the physical distance between processing, memory and switching modules grows.
Overcoming Connectivity Bottlenecks
Astera Labs delivers semiconductor-based connectivity solutions purpose-built to unleash the full potential of intelligent data infrastructure at cloud-scale. To date, we’ve developed three class-defining, first-to-market product lines that deliver critical connectivity for high-value artificial intelligence and machine learning applications.
Our connectivity solutions enable disaggregated, heterogeneous Generative AI architectures that reduce cost and power compared to optical solutions. Our Aries Smart Retimers extend the reach for PCIe® NICs, GPUs, FPGAs, and NVMe drives within servers. Our Leo Memory Connectivity Platform enables CXL®-attached memory expansion, pooling, and sharing for cloud servers. And, our Taurus Smart Cable Modules are optimal for Ethernet connectivity for switch-to-switch and switch-to-server topologies. We’ve also included support for fleet management capabilities and health monitoring diagnostics to predict failures before they happen and to increase multi-tenant AI system performance and uptime, ease re-provisioning, and lower total cost of ownership (TCO).
Learn more about our intelligent connectivity solutions:
- Aries PCIe®/CXL® Smart Retimers enable generative AI applications by improving signal integrity, extending reach up to 3x and supporting high bandwidth communication between GPUs or accelerators required to manage the complex datasets involved in training the large language models.
- Leo CXL Memory Connectivity Platform eliminates memory bandwidth and capacity bottlenecks by enabling up to 2TB more memory per CPU. Leo is optimized for meeting complex computational needs of generative AI workloads at low latency.
- Taurus Ethernet Smart Cable Modules™ remove rack-level ethernet bottlenecks by overcoming reach, signal integrity, and bandwidth utilization issues in 100G/Lane Ethernet Switch-to-Switch and Switch-to-Server applications. By enabling thinner cables and supporting gearboxing and breakouts, these modules are optimized for high-density, high-throughput AI/ML rack configurations.
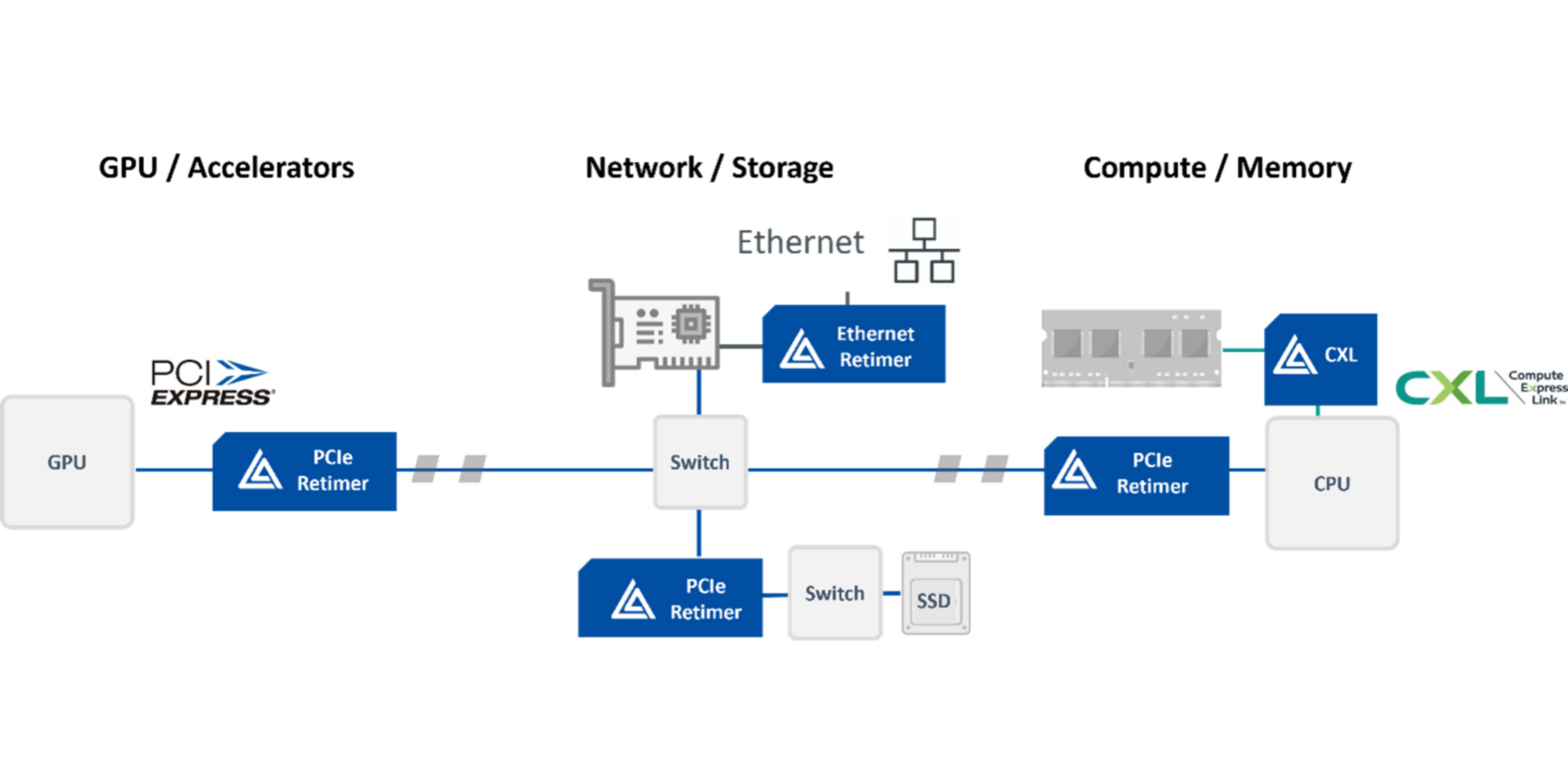
Accelerating Time-to-Market
At Astera Labs, we understand that supporting plug-and-play interoperability is critical for deploying these new architectures at scale. In our industry-first Cloud-Scale Interop Lab, we rigorously test our portfolio for standards compliance and system-level interoperation with all major hosts, endpoints, and memory modules – so our customers can deploy with confidence and accelerate time-to-market.
Conclusion
The Age of AI is here, and Astera Labs’ intelligent connectivity solutions for data infrastructure can help you seize the booming Generative AI opportunity. Contact us to learn more.
References